If science were a dating app, quantum physics and machine learning probably wouldn’t be a match. They’re from completely different fields and often require completely different backgrounds and skills. But, throw in a little quantum computing and, suddenly, that science-matchmaking app becomes Tinder and the attraction between the two is palpable.
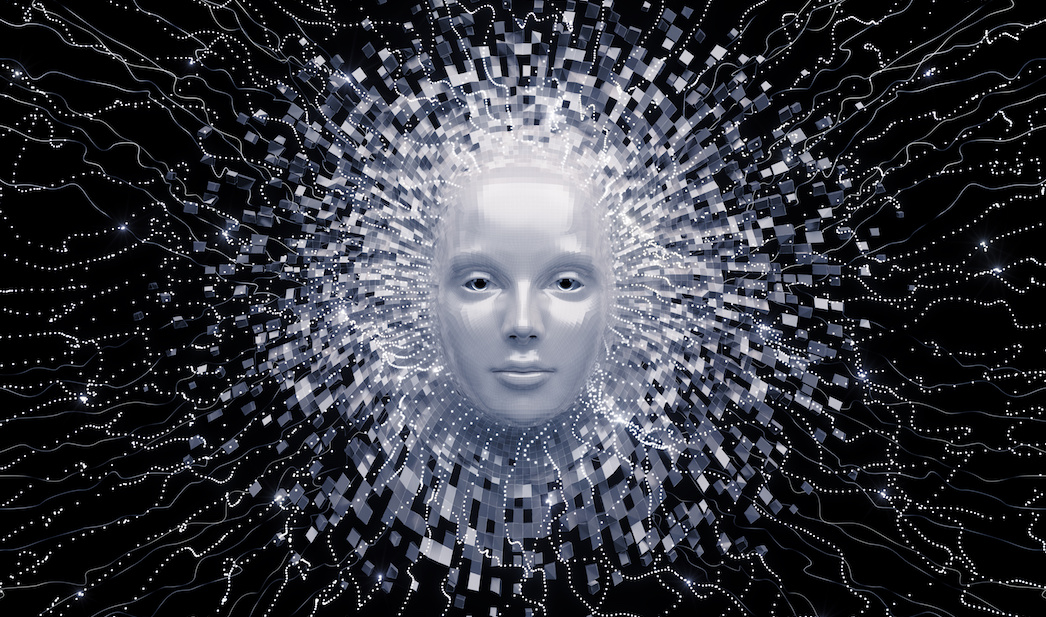
(Credit: cmo.adobe.com/articles/2017/5/how-will-artificial-intelligence-impact-business-tlp-ptr.html#gs.5zlifl)
Even though the extent of change that quantum computing will unleash on AI is up for debate, many experts now more than suspect that quantum computing will definitely alter AI at some level. Analysts from bank holding company BBVA, for example, point toward the natural synergy between quantum computing and AI as reasons why quantum machine learning will eventually best classical machine learning.
“Quantum machine learning can be more efficient than classic machine learning, at least for certain models that are intrinsically hard to learn using conventional computers,” says Samuel Fernández Lorenzo, a quantum algorithm researcher who collaborates with BBVA’s New Digital Businesses area. “We still have to find out to what extent do these models appear in practical applications.”
Here are four ways quantum computers could change the future of AI. Forever.
1. Handling HUGE Amounts of Data
Machine learning and AI eat data. Lots of data. Quantum computers are designed to manage huge amounts of data. With each iteration of quantum computer design and improvements to quantum error-correction code, programmers are able to better master the potential of qubits — quantum bits — to manage exponentially more data, according to Lorenzo.
“In classical computing we know how to solve problems thanks to computer language (AND, OR NOT) used when programming,” said Lorenzo. “Operations that are not feasible in bit computing can be performed with a quantum computer. In a quantum computer all the numbers and possibilities that can be created with N qubits are superimposed (if there are 3 qubits, there will be 8 simultaneous possible permutations.) With 1,000 qubits the exponential possibilities far exceed those that we have in classical computing.”
2. Building Better Models
Several industries, such as pharmaceutical, life sciences and finance, are nearly at the end of their classical computing rope. These industries require complex models that classical computers just can’t generate. Quantum computers, on the other hand, have the potential processing power to model the most complex situations. If quantum technology can create better models, it may lead to better treatments for disease, decreased risk of financial implosions, and improved logistics.
3. More Accurate Algorithms
According to Lorenzo, supervised learning is used for most industrial applications of artificial intelligence, such as image recognition or consumption forecasting. Quantum Machine Learning — QML — researchers are trying to find ways to develop better quantum computer algorithms.
“In this area, based on the different QML — quantum machine learning — proposals that have already been set forth, it is likely that we’ll start seeing acceleration – which, in some cases, could be exponential – in some of the most popular algorithms in the field, such as ‘support vector machines’ and certain types of neural networks,” explains Fernández Lorenzo.
Quantum computing should have an immediate impact on traditional AI models and algorithms, such as non-supervised learning and reinforcement learning, according to the researcher.
“Dimensionality reduction algorithms are a particular case. These algorithms are used to represent our original data in a more limited space, but preserving most of the properties of the original dataset,” said Lorenzo.
He added that quantum computing’s particular skill will help pinpoint certain global properties in a dataset, not so much specific details…
Reinforcement learning is an AI model that is used to handle complex situations, for example, in videogaming, but many experts suggest that the model’s potential is much greater. The most demanding task here, in terms of computing workload and time consumption, is training the algorithm.“In this context, some theoretical proposals have already been laid out to accelerate this training using quantum computers, which may contribute to developing an extremely powerful artificial intelligence in the future,” said Lorenzo.
4. Using Multiple Datasets
The problem often isn’t that there is not enough data, or that there’s too much data, the problem is that the data is placed in a variety of datasets, according to futurist and strategic adviser Bernard Marr. He writes that quantum computers could handle the integration of different datasets for much quicker and easier analysis.
“The promise is that quantum computers will allow for quick analysis and integration of our enormous data sets which will improve and transform our machine learning and artificial intelligence capabilities,” he writes.
AI and Quantum Collaborations Are Happening Now
The natural extension that quantum computers offer machine learning and artificial intelligence is not lost on entrepreneurs, who are busy now learning ways to exploit the technical combination. Recently, The Quantum Daily reported on a deal that was signed between C-DAC and Atos, two companies who are investigating the match between quantum computing and AI.