Извлечение информации означает создание структурированных данных из неструктурированного текста. На практике задача может выглядеть так: нужно автоматически создать запись в календаре исходя из текста письма, как на рисунке ниже.
User
Простым языком о языковых моделях и цепи Маркова (Markov Chain)
N-граммы
N-граммы – это статистические модели, которые предсказывают следующее слово после N-1 слов на основе вероятности их сочетания. Например, сочетание I want to в английском языке имеет высокую вероятностью, а want I to – низкую. Говоря простым языком, N-грамма – это последовательность n слов. Например, биграммы – это последовательности из двух слов (I want, want to, to, go, go to, to the…), триграммы – последовательности из трех слов (I want to, want to go, to go to…) и так далее.
Такие распределения вероятностей имеют широкое применение в машинном переводе, автоматической проверке орфографии, распознавании речи и умном вводе. Например, при распознавании речи, по сравнению с фразой eyes awe of an, последовательность I saw a van будет иметь большую вероятность. Во всех этих случаях мы подсчитываем вероятность следующего слова или последовательности слов. Такие подсчеты называются языковыми моделями.
Как же рассчитать P(w)? Например, вероятность предложения P(I, found, two, pounds, in, the, library). Для этого нам понадобится цепное правило, которое определяется так:
Detecting attempts of mass influencing via social networks using NLP. Part 2
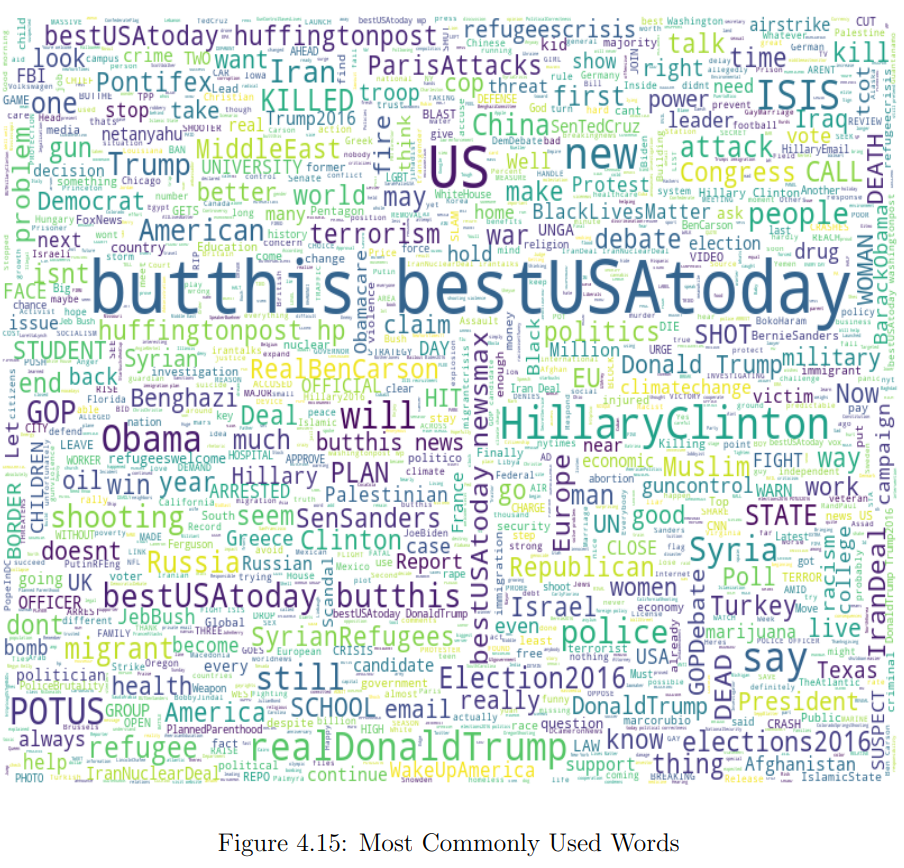
In Part 1 of this article, I built and compared two classifiers to detect trolls on Twitter. You can check it out here.
Now, time has come to look more deeply into the datasets to find some patterns using exploratory data analysis and topic modelling.
EDA
To do just that, I first created a word cloud of the most common words, which you can see below.
Detecting attempts of mass influencing via social networks using NLP. Part 1
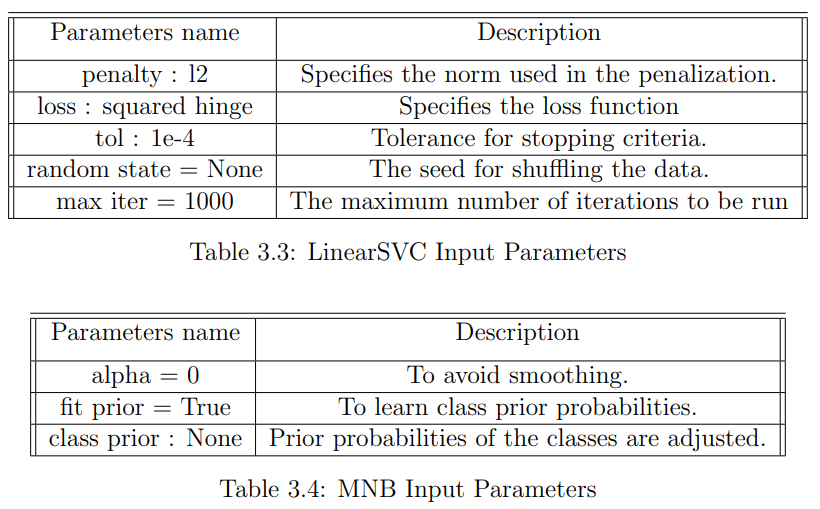
During the last decades, the world’s population has been developing as an information society, which means that information started to play a substantial end-to-end role in all life aspects and processes. In view of the growing demand for a free flow of information, social networks have become a force to be reckoned with. The ways of war-waging have also changed: instead of conventional weapons, governments now use political warfare, including fake news, a type of propaganda aimed at deliberate disinformation or hoaxes. And the lack of content control mechanisms makes it easy to spread any information as long as people believe in it.
Based on this premise, I’ve decided to experiment with different NLP approaches and build a classifier that could be used to detect either bots or fake content generated by trolls on Twitter in order to influence people.
In this first part of the article, I will cover the data collection process, preprocessing, feature extraction, classification itself and the evaluation of the models’ performance. In Part 2, I will dive deeper into the troll problem, conduct exploratory analysis to find patterns in the trolls’ behaviour and define the topics that seemed of great interest to them back in 2016.
Features for analysis
From all possible data to use (like hashtags, account language, tweet text, URLs, external links or references, tweet date and time), I settled upon English tweet text, Russian tweet text and hashtags. Tweet text is the main feature for analysis because it contains almost all essential characteristics that are typical for trolling activities in general, such as abuse, rudeness, external resources references, provocations and bullying. Hashtags were chosen as another source of textual information as they represent the central message of a tweet in one or two words.
Autosupply или как автоматизировать цепочки поставок с помощью ML
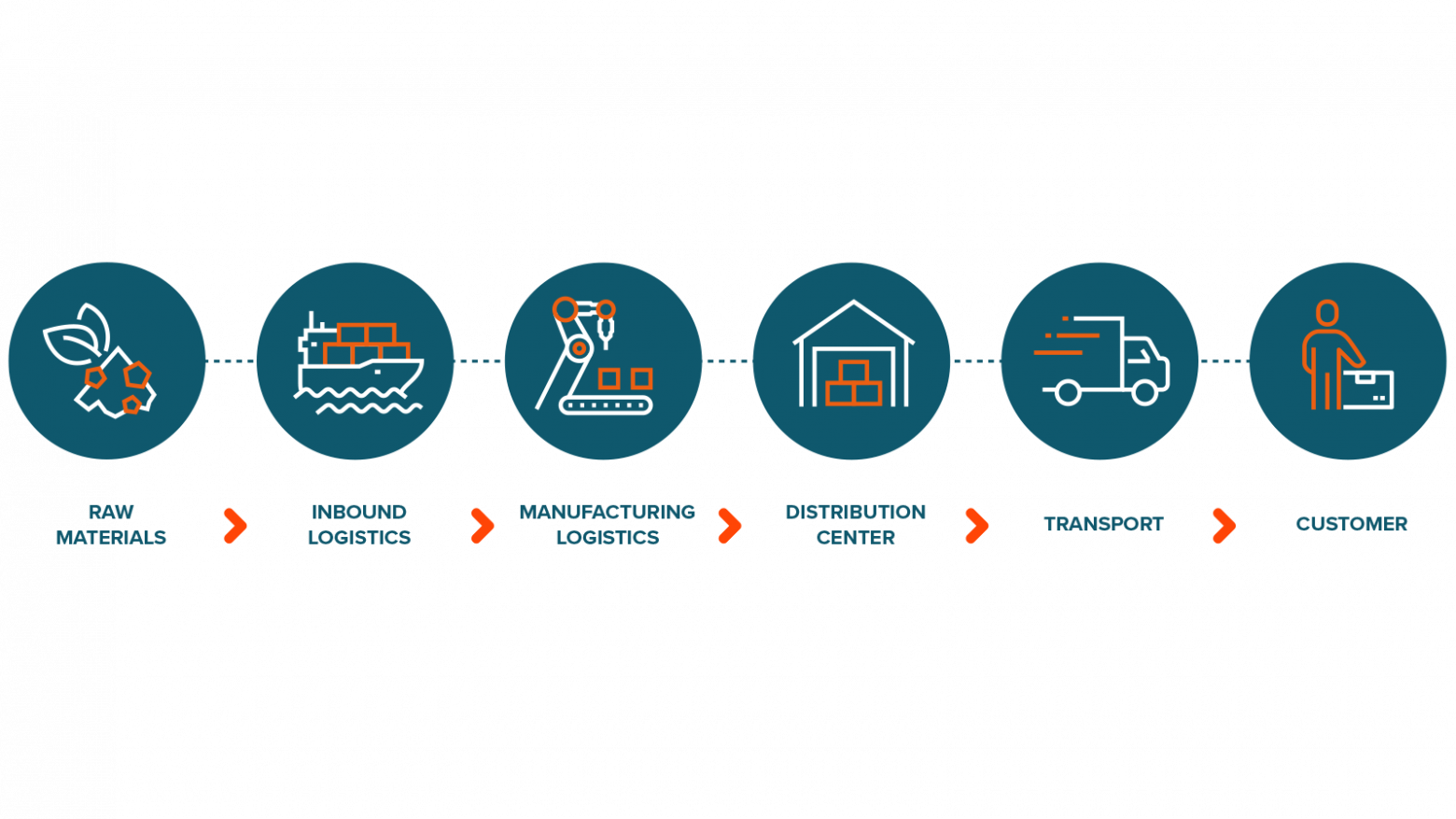
В этой статье речь пойдет о предиктивном определении поставки товарно-материальных ценностей в сеть фронт-офисов банка. Проще говоря, об автоматизированной организации снабжения отделений бумагой, канцтоварами и другими расходными материалами.
Этот процесс называется автопополнение и состоит из следующих этапов – прогнозирование потребности в центре снабжения, формирование заказа там же, согласование и корректировка потребности розничным блоком и непосредственно поставка. Слабое место здесь – необходимость ручной корректировки и последующего согласования объема поставки менеджерами логистики и руководителями подразделений.
Какой этап в этой цепочке можно оптимизировать? Во время формирования заказа менеджеры логистики рассчитывают количество товаров к поставке, основываясь на ретро-данных, данных о срочных заказах и своем экспертном опыте. При этом руководители отделений, чтобы обосновать потребность в тех или иных товарах, должны отслеживать их расход и понимать текущие запасы в отделении. Если мы научимся определять точную потребность в товарах и автоматизируем этот расчет, то этапы формирования и корректировки заказа будут занимать гораздо меньше времени или даже станут вовсе не нужны.
Задача прогнозирования потребления
Есть очень похожая и более распространенная задача в розничной торговле: сколько каких товаров нужно поставить в магазин Х в момент времени У? Задача решается относительно просто: зная потребление товара во времени из чеков и запасы товара на складе, можно вычислить будущую поставку напрямую. Поставить нужно столько, сколько предположительно продадут, за минусом запаса.
How we tackled document recognition issues for autonomus and automatic payments using OCR and NER
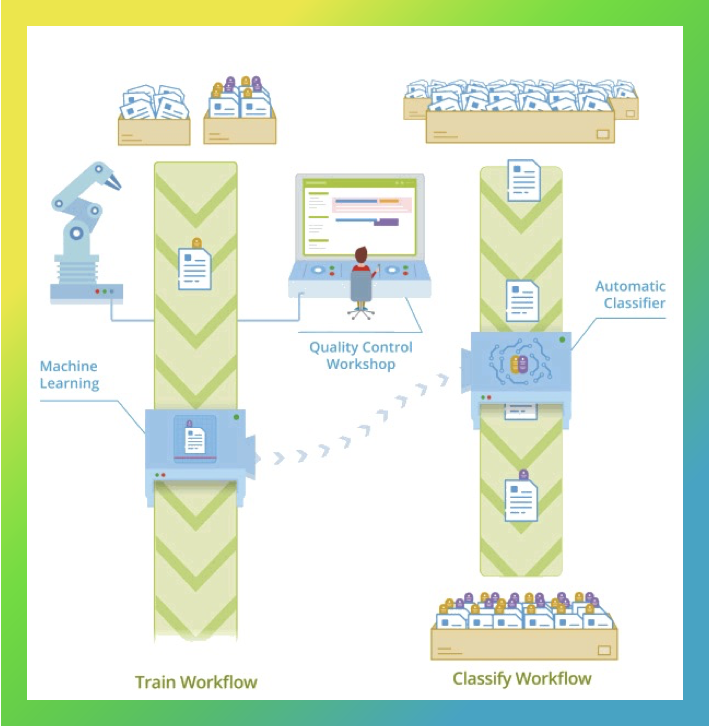
In this article, I would like to describe how we’ve tackled the named entity recognition (aka NER) issue at Sber with the help of advanced AI techniques. It is one of many natural language processing (NLP) tasks that allows you to automatically extract data from unstructured text. This includes monetary values, dates, or names, surnames and positions.
Just imagine countless textual documents even a medium-sized organisation deals with on a daily basis, let alone huge corporations. Take Sber, for example: it is the largest financial institution in Russia, Central and Eastern Europe that has about 16,500 offices with over 250,000 employees, 137 million retail and 1.1 million corporate clients in 22 countries. As you can imagine, with such an enormous scale, the company collaborates with hundreds of suppliers, contractors and other counterparties, which implies thousands of contracts. For instance, the estimated number of legal documents to be processed in 2022 has been over 65,000, each of them consisting of 30 pages on average. During the lifecycle of a contract, a contract usually updated with 3 to 5 additional agreements. On top of this, a contract is accompanied by various source documents describing transactions. And in the PDF format, too.
Previously, the processing duty befell our service centre’s employees who checked whether payment details in a bill match those in the contract and then sent the document to the Accounting Department where an accountant double-checked everything. This is quite a long journey to a payment, right?
Information
- Rating
- Does not participate
- Registered
- Activity