A/B test is not enough
There is a common opinion that A/B test is a universal, half-automatic tool that always helps to increase conversion, loyalty and UX. However misinterpretation of results or wrong sampling leads to the loss of loyal audience and decrease of margin. Why? A/B is based on the basic assumption that this sample is homogeneous and representative, scalability of results. In reality, the audience is heterogeneous — recall the “20/80” distribution for income. Heterogeneity means that sensitivity to A/B varies significantly within the sample.
Audience clustering is a real effect (rule, not exception according to Pareto), which means the presence of different psychological profile groups of clients in one pool. Evaluation of conversion confidence interval implies uniformity. Therefore, violation of these criteria means that the accuracy of results is immeasurable. Result without accuracy is garbage. Each unique psychological profile reacts with different sensitivity to the campaign or feature. We assume that a profile is a unique set of features. For simplicity, two profile sets X and Y may be considered. Some features of several profiles may intersect – your girlfriend loves coffee and chocolate as well. Let us illustrate this effect in form of three topologies:
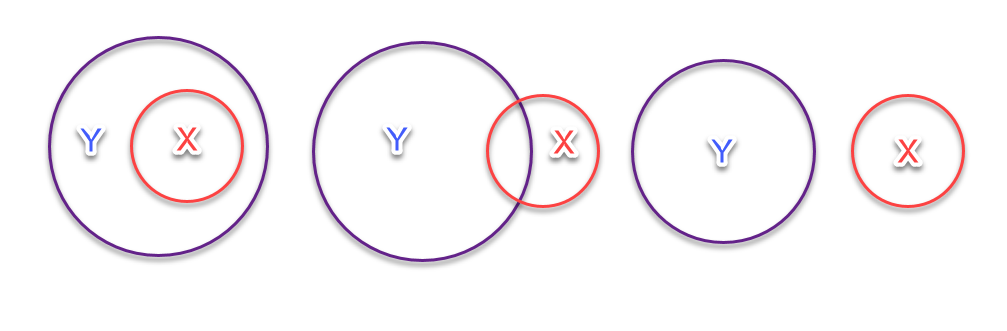
By default, we assume that we cover all segments at once — Сase I. Case II and III involve non-trivial scenarios. Consider a typical scenario of Сase II. Conversion increased significantly – Y set shows a positive reaction, while X gave a negative reaction and negative NPS change. Y set is larger in the random sample with no weights, so the cumulative effect is positive. Conversion increased twice. Now imagine that the average check of X is 10 times higher and the conversion of segment X has fallen by half. Finally: increase of conversion, loss of audience, profit decline. The problem is aggravated by intuitive tricks. Sometimes automotive models tests the hypothesis on segment X (Сase III) and try to generalize to the union (X + Y). What is wrong? The sampling technique does not take into account segmentation. Solutions?
- Way # 1. Cluster the audience using k-means, other ML models, or RFM analysis. You need to know the hyperparameter — the number of groups as the input. Its definition is not trivial. The next step is to determine individual conversion of the segment. Personalize the campaign — offer A or B script, depending on profile.
- Way # 2. Measure A/B margin. Recall that margin is the product of conversion, traffic, and the average price. The last two parameters can be fixed by selecting a separate category of goods and choosing a uniform traffic period – slow parameters. You may increase the discreteness of traffic measurement (every Monday for a month) to reduce the random component.
- Way # 3. Stability analysis. Sampling with replacement is used in this case. All segments are considered. The sample size is gradually increased. Log-Log representation of conversion vice sample size gives the regression slope (Hurst factor). It provides understanding of uniformity and renorm stability.
However. No matter what path you choose, the audience will change with higher frequency. This means that the A/B test is a regularly repeated experiment. An experiment that should be supervised by an experienced analyst despite a significant number of commercial automated solutions. Do not forget that all models are wrong, but some are temporarily useful…under certain conditions.
Dedicated to my father who taught me that intuition is just as important as math